Graficando y midiendo el impacto de la primera ola de COVID-19 en la economía boliviana: enfrentando lo desconocido
DOI:
https://doi.org/10.35319/lajed.202136451Palabras clave:
COVID-19, Análisis de series de tiempo interrumpidas, Modelos ARMA-GARCH, BoliviaResumen
El índice mensual de actividad económica boliviano, junto a modelos ARMA, es utilizado en un intento de graficar y medir el impacto de la pandemia COVID-19 sobre la economía boliviana. La diferencia acumulada entre los valores observados y contrafactuales muestra una pérdida global de 12.6% de actividad económica en los diez meses, de febrero a noviembre de 2020, de la primera ola de COVID-19, con una recuperación de corto plazo con forma de W inclinada justo antes del inicio de la segunda ola, en diciembre de 2020. El desglose de la economía en sus 12 sectores muestra amplia heterogeneidad en la profundidad del impacto y velocidades de recuperación durante el mismo periodo.
Descargas
Citas
Bollerslev, T. (1986). Generalized autoregressive conditional heteroscedasticity. Journal of Econometrics, (31), 307-327.
Box, G.E.P. and Jenkins, G.M. (1970). Time series analysis, forecasting and control. San Fransisco: Holden-Day.
Box, G.E.P., Jenkins, G.M. and Reinsel, G.C. (1994). Time series analysis, forecasting and control (3rd ed.). Prentice-Hall.
COVID-19 data (2020). Bolivia Segura, Estado Plurinacional de Bolivia. https://www.unidoscontraelcovid.gob.bo/index.php/category/reportes/
Craig, P., Cooper, C., Gunnell, D., Haw, S., Lawson, K., Macintyre, S., Ogilvie, D., Petticrew, M., Reeves, B., Sutton, M. and Thompson, S. (2012). Using natural experiments to evaluate population health interventions: new Medical Research Council guidance. Journal of Epidemiology & Community Health,66(12). 1182-1186. http://dx.doi.org/10.1136/jech-2011-200375
Engle, R.F. (1982). Autoregressive conditional heteroscedasticity with estimates of the variance of United Kingdom inflation. Econometrica, 50(4), 987-1007.
Hudson, J., Fielding, S. and Ramsay, C. (2019). Methodology and reporting characteristics of studies using interrupted time series design in healthcare. BMC Medical Research Methodology, 19. https://bmcmedresmethodol.biomedcentral.com/articles/10.1186/s12874-019-0777-x
IGAE data (2008-2020). Instituto Nacional de Estadística (INE). La Paz. https://www.ine.gob.bo/index.php/estadisticas-economicas/indice-global-de-actividad-economicaigae/
Schaffer, A.L., Dobbins, T.A. and Pearson, S.A. (2021). Interrupted time series using autoregressive integrated moving average (ARIMA) models: a guide for evaluating large-scale health interventions. BMC Medical Research Methodology, 21. https://bmcmedresmethodol.biomedcentral.com/track/pdf/10.1186/s12874-021-01235-8.pdf
Williams, J.C. (2017). The perennial problem of predicting potential. Federal Reserve Bank of San Francisco Economic Letter 2017-32. https://www.frbsf.org/economicresearch/files/el2017-32.pdf
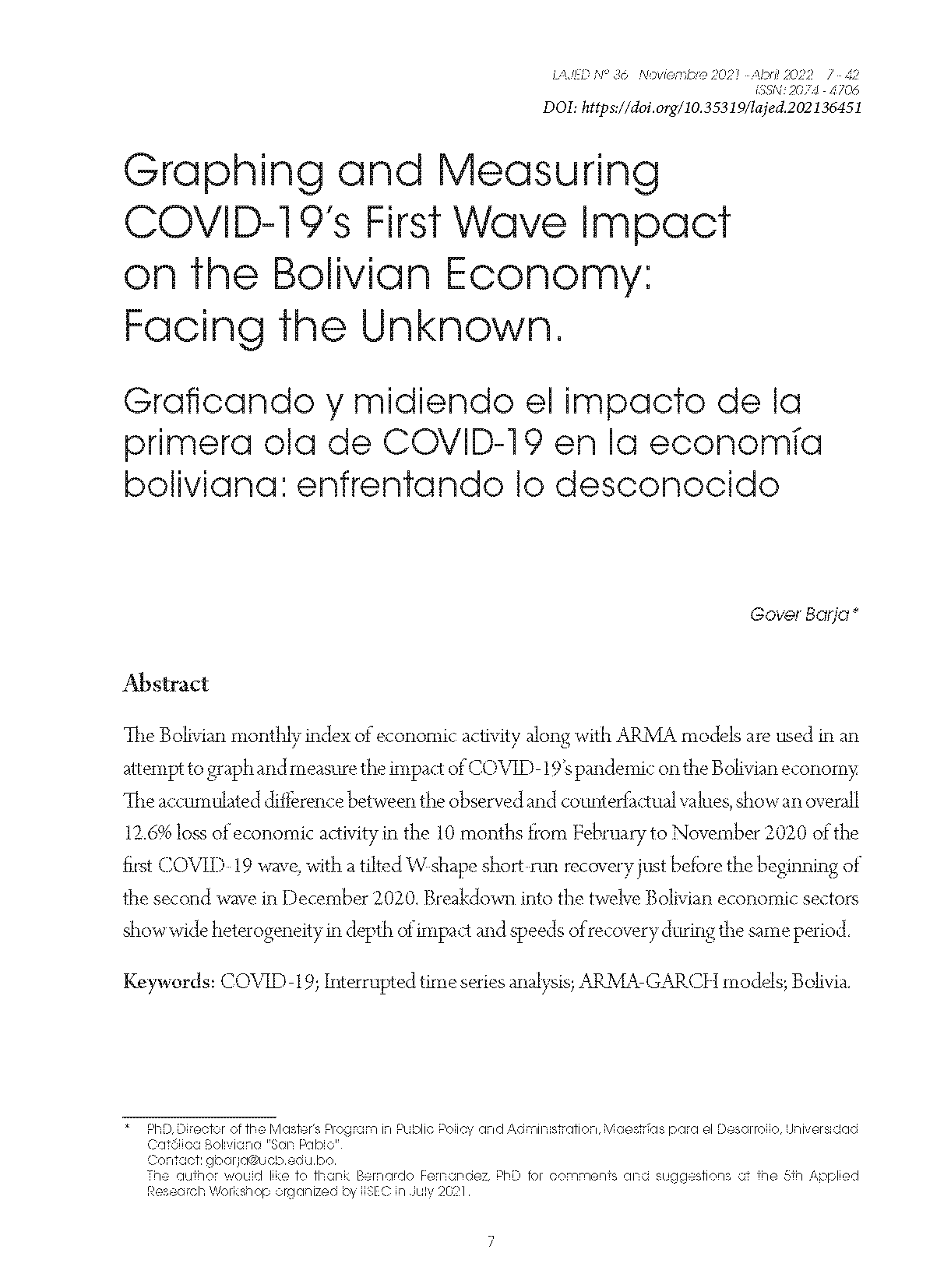